How Life Edit is using AI to expand the therapeutic boundaries of CRISPR

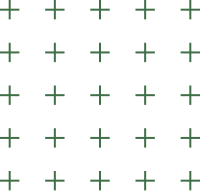
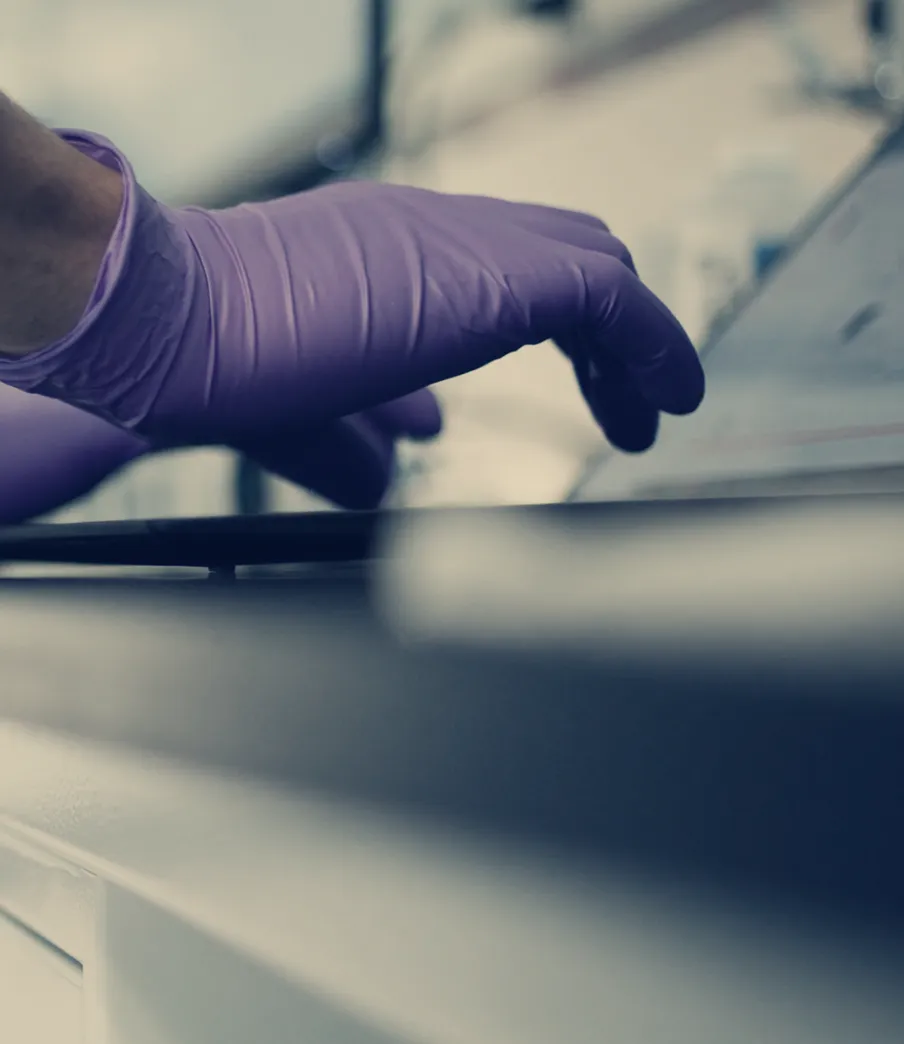
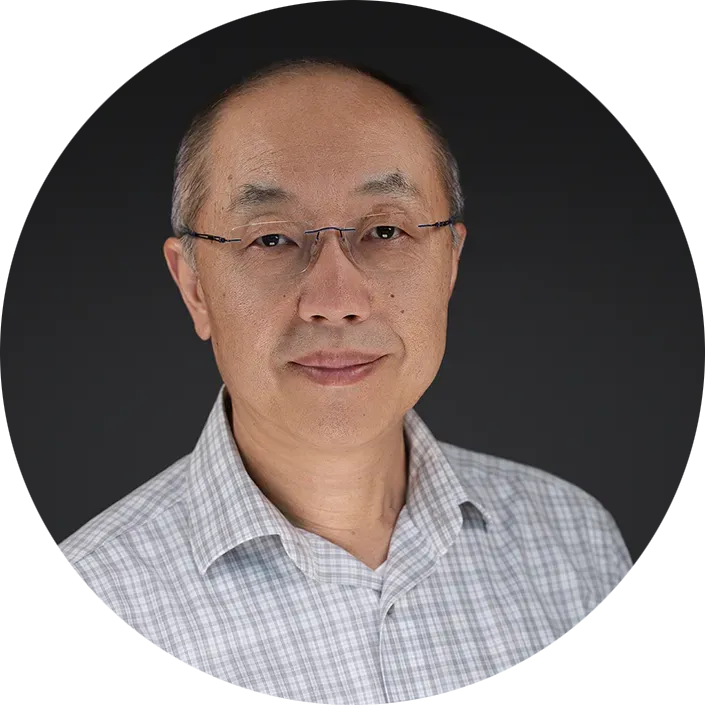
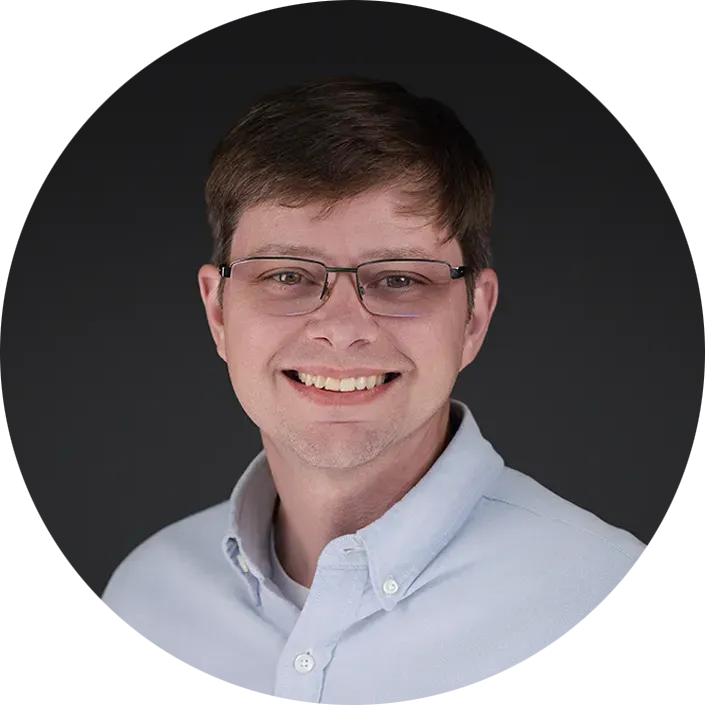
The recent convergence of artificial intelligence (AI) and biology has spurred remarkable scientific advances, most notably AlphaFold’s ability to predict 3D structures of proteins from amino acid sequences. These advances have been fueled by the availability of data for training AI models and technology capable of processing data faster than ever before, and they demonstrate the power of AI to revolutionize biological research.
Gene editing technologies, especially CRISPR, are likewise revolutionizing the therapeutic landscape with the promise of curing diseases in a single dose by correcting their underlying genetic drivers. Given the myriad diseases that CRISPR could treat, AI is becoming indispensable for delivering on the promise of gene editing.
Life Edit, ElevateBio’s gene editing business, is uniquely positioned at the interface of computational science and CRISPR biology to do just that. Here, we examine why AI is essential to the future of CRISPR discovery, what challenges AI faces in the CRISPR development, and how Life Edit is addressing those challenges to accelerate CRISPR discovery and enable our partners to develop novel and life-changing genetic medicines.
AI is indispensable for future CRISPR gene therapies
The first wave of CRISPR therapies have focused on a small collection of monogenic diseases, such as sickle cell disease, where the underlying cause is a single SNP in a single gene that can be corrected with ex vivo editing of patients’ cells. Developing CRISPR systems to treat these diseases, without the aid of AI, is relatively straightforward with current experimental approaches. The real challenge lies in expanding CRISPR’s application to the thousands of more complex monogenic disorders, as well as the multitude of polygenic diseases involving multiple gene corrections. Treating all these diseases requires development of many custom CRISPR systems, which would take an extremely long time to achieve with conventional approaches.
A key barrier with conventional approaches is that – for reasons researchers do not fully understand – different CRISPR systems are often needed to edit different disease-associated loci; successful gene editing for each disease thus involves the lengthy process of engineering and optimizing both the nuclease and a guide RNA (gRNA) of a given CRISPR system. There is no quick and rational way to effectively predict and design a CRISPR system with the optimal editing window, potency and specificity for a disease – despite the large quantities of experimental data available in the literature and proprietary databases.
These databases are insufficient for the task because the spaces of possible CRISPR protein sequences (based on 20 amino acid letters) and possible RNA sequences (based on four nucleotide letters) are astronomically huge and cannot be fully explored by experimental means alone. We believe AI is well positioned to tackle this problem using large language models (LLMs). Our belief is encouraged by recent AI advances in small molecule drug discovery, where AI models, trained on high throughput screening data, can perform virtual screening to rapidly narrow the number of hits for experimental validation.
Training AI in the language of therapeutics
While LLMs such as ChatGPT represent a major breakthrough in AI’s ability to use everyday human language, AI models for CRISPR nucleases are not quite as developed. Current AI models trained on natural CRISPR sequences from public databases are capable of predicting CRISPR structures and even generating novel systems. However, upon experimental validation, many do not have sufficient editing activity in mammalian cells, and those that do have sufficient activity sometimes perform at a lower level of efficiency than the current benchmark nuclease, SpyCas9. We believe the gap between function and therapeutic utility might exist because bacteria evolved CRISPR to detect and destroy infecting viruses, not edit and correct human genes, so there is no evolutionary pressure to produce CRISPR systems that are efficient in mammalian cells for treating diseases.
To achieve AI models that have therapeutic utility, we need to train the models with a large amount data from wet-lab experiments that are specifically designed for improving editing activity and specificity in disease-relevant mammalian cells. These data should include sufficient information of systematic search in the CRISPR protein and RNA sequence space that can address potential bottlenecks in gene editing inside mammalian cells, which are probably multifaceted and cell- or disease-dependent. AI models can help us find the right systems for the right targets quickly, without having to understand all of the bottlenecks, and these models should be continuously improved by a feedback loop, in which model predictions are constantly evaluated by experiments. Only with therapeutically relevant AI models for CRISPR we will be able to adequately address the challenges of developing CRISPR systems for the vast majority of monogenic and polygenic diseases.
Life Edit’s solutions for bridging the CRISPR gap
Life Edit is an integral part of ElevateBio’s genetic medicine ecosystem of enabling technologies and end-to-end manufacturing capabilities. We’re also distinct in how we work compared to other gene editing companies. We started with the “top-down” approach of building a diverse array of CRISPR nucleases and base editors suitable for treating a wide range of diseases differentiates us in several ways. Now we are focused more on the “bottom-up” approach of discovering and developing CRISPR systems to treat select diseases for our partners and our own therapeutic development programs.
First, we’re not just working with state-of-the-art computation and AI: we’re pushing their boundaries. We know this, because the questions we’re asking some of the technology sector’s AI leaders are stretching their capabilities into new areas.
Second, we have collected and curated our own proprietary protein database, which constitutes a heterogeneous collection of sequences from isolated organisms and metagenomes. The collection contains proteins from large public collections and numerous published studies, along with proprietary sequences from diverse biomes. These data have been unified, annotated, and structured to promote model development toward protein engineering.
Third, we are generating a large body of experimental data, tailored to the therapeutic needs CRISPR aims to address. We’re using these data to build and train AI models that can predict and generate CRISPR systems, customized to the target disease sequences with high activity and specificity. These AI-generated systems should have significantly higher success rates as gene editing therapies than natural systems and could broaden the disease areas treatable with CRISPR.
Finally, the integration of our computational and experimental labs enables a seamless transition for prediction to experimental validation of the AI-generated CRISPR systems. Our structure allows our computational and experimental teams to work hand in hand, framing questions for our AI models in terms of how the output could be tested experimentally. We want to know: Is the model really gaining us something? Is this something we want to do again? We’re testing whether AI-based recommendations are better than human recommendations – something the field believes should be true but hasn’t yet proven generally.
With our AI CRISPR models, Life Edit can serve not only the needs of our own pipeline, but the whole field of CRISPR gene therapy as well through partnerships. With our models and portfolio of validated CRISPR systems, we are the first stop for a company starting a CRISPR gene therapy program; with our enabling technologies and the end-to-end manufacturing capabilities of ElevateBio’s BaseCamp, we are a “one-stop shop” and ideal partner for developing a CRISPR drug.
We can offer partners and clients many flexible options, not just one, for developing and commercializing their gene editing therapies, thus putting more shots on goal as an industry than any one organization could otherwise pursue independently. We are propelling the entire CRISPR gene editing field into the future, to bring these potentially curative medicines to as many patients as possible.